According to Techreport SAP ERP systems are utilized by over 400,000 organizations worldwide spanning various industries. Notably, about 86% of Fortune 500 companies and 92% of Forbes Global 2000 companies are SAP customers. With an estimated 77% of global transaction revenue passing through SAP systems, the platform’s influence is significant.
Organizations using SAP have numerous options when it comes to data analytics and cloud solutions, especially when comparing SAP's native analytics tools (e.g., SAP BW/4HANA, Datasphere, SAP Analytics Cloud) with hyperscaler offerings like AWS, Microsoft Azure (Fabric), and Google Cloud. Deciding between these platforms is crucial for optimizing an organization’s analytics strategy, with each providing unique advantages depending on specific business requirements.
To evaluate these platforms, we will explore the following areas:
Data Management and Sources
Reporting, EPM and Analytics
Infrastructure Scalability
Frontend Preferences and Limitations
Cost and Development
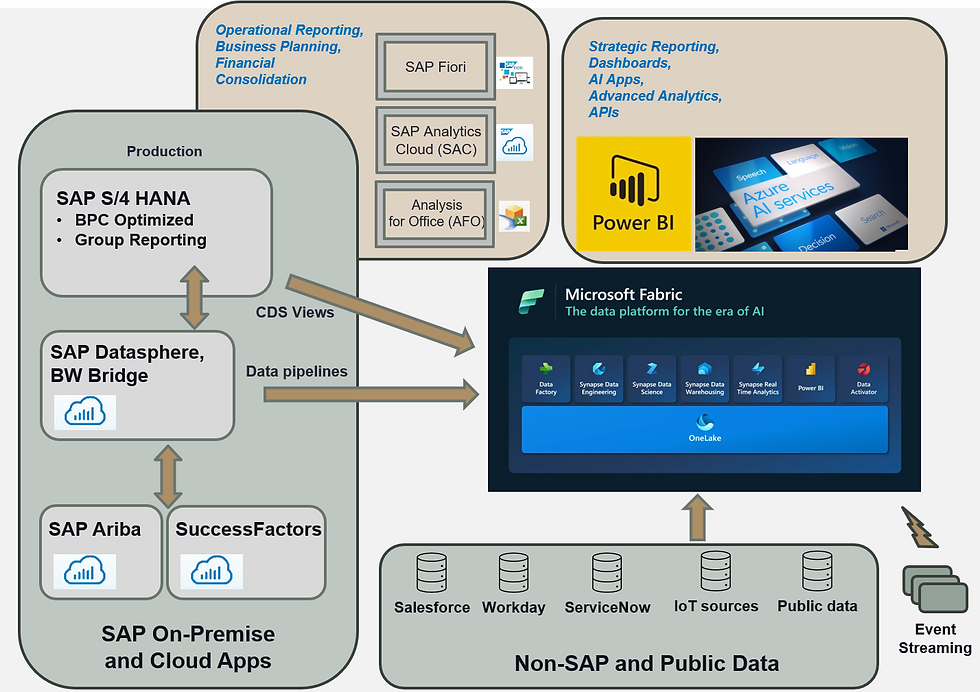
1. Data Management and Sources
Choosing the right analytics platform often starts with understanding your data sources and structures.
Data sources: SAP vs. Cloud apps or public data
SAP Analytics tools and capabilities provide a seamless connection for organizations with most of the data residing in SAP systems such as SAP S/4HANA. On the other hand, hyperscalers might be the preferred choice when integrating non-SAP applications like Salesforce, Workday, Magento, ServiceNow, or other cloud services and public datasets.
Non-structured, semi-structured data sources
SAP customers frequently leverage a wide range of public data sources, such as government open data portals, financial data from platforms like Yahoo Finance and Bloomberg, and geospatial data from providers like OpenStreetMap and NOAA. These datasets are typically integrated into analytics platforms to enhance business decision-making across areas like economic forecasting, sustainability, and supply chain management.
Other non-SAP data commonly used in organizations include IoT logs, social media feeds, market research, and consumer insights from providers like Nielsen, etc. These public and external datasets are often delivered in semi-structured formats like JSON, XML, and CSV, offering flexibility in storage and analysis.
While hyperscalers excel in handling unstructured and semi-structured data using their advanced big data and data lake architectures, SAP Analytics primarily focuses on efficiently processing structured data from enterprise systems.
2. Reporting, EPM and Analytics
Different reporting needs and analytics capabilities dictate the optimal platform for your business.
Operational vs Strategic reporting
SAP Analytics is best suited for operational reporting, especially for real-time insights from ERP systems. In SAP S/4HANA, for example, numerous built-in analytics Fiori apps can be used out of the box for operational reporting.
Hyperscalers with their ability to handle massive datasets, on the other hand, are ideal for strategic reporting that may involve long-term analysis from various data sources.
Real-time vs Batch loads
SAP’s in-memory HANA technology supports real-time analytics for critical decision-making, especially when used directly on the SAP S/4HANA platform. Hyperscalers, however, are more flexible for handling batch processing, which is necessary for large data volumes that don’t require instant insights.
Enterprise Performance Management
If your organization requires enterprise-wide planning and consolidation functionalities, SAP Analytics Cloud (SAC), SAP BPC, and/or SAP Group Reporting integrate natively with SAP’s financial and operational planning tools, making it a powerful solution for FP&A, business planning and financial consolidation needs.
At the same time, non-SAP EPM applications (e.g. Anaplan, Tagetik, OneStream) have gained popularity and can integrate with both SAP data platforms and hyperscalers. Their integration with SAP is typically managed through either SAP connectors or via APIs, middleware, or third-party connectors.
Anaplan, for example, excels in connected planning across multiple business functions, offering flexibility and scalability with a user-friendly interface. It tends to be favored for non-SAP environments with its strong collaborative planning features, while SAC/BPC excels for SAP-centric organizations needing seamless integration with existing SAP data and workflows.
AI, ML capabilities
When it comes to AI and machine learning, hyperscalers like AWS SageMaker, Google AI, and Azure Machine Learning are widely recognized for their extensive machine learning frameworks, which provide advanced tools for data scientists to build, train, and deploy models. These platforms are favored for their flexibility, scalability, and a broad range of machine learning services, allowing for complex and large-scale AI initiatives.
In contrast, SAP offers more specific, integrated AI and machine learning capabilities tailored to its ecosystem. The SAP Business Technology Platform (BTP) includes SAP AI Core, SAP AI Business Services, and SAP Data Intelligence, which allow organizations to automate data management, build custom ML models, and deploy pre-built AI services like document processing, predictive analytics, and chatbots. SAP’s AI tools are deeply integrated with SAP S/4HANA and other SAP applications, facilitating real-time insights and intelligent automation within existing SAP-driven business processes.
3. Infrastructure Scalability
Scalability is a key factor when handling large volumes of data or spikes in usage.
Scalability & Performance
Hyperscalers such as AWS, Azure, and Google Cloud provide virtually unlimited scalability, making them the better option for workloads that require flexible and elastic scaling. SAP Analytics, while scalable, is typically more suitable for SAP-heavy environments where seamless integration with SAP applications is a priority.
Infrastructure support
Hyperscalers offer a wider range of infrastructure options, including serverless architectures, auto-scaling, and a broader set of tools for managing and scaling complex workloads across global data centers. Infrastructure support is usually provided by the vendor and included in the price.
For supporting SAP Analytics infrastructure you normally need to have an infrastructure team focusing on network connectivity, infrastructure installation, support, patching, security, and authorizations.
Deploying SAP Analytics tools can take anywhere from several weeks to several months, depending on factors such as infrastructure (on-premise vs. cloud), data integration complexity, customization, and user training. Cloud-based solutions like SAP Analytics Cloud and Datasphere require less time and effort, while on-premise tools like SAP BW/4HANA can involve more extensive infrastructure and setup efforts.
4. Frontend Preferences and Limitations
How users interact with data is a crucial element of your decision-making process.
Frontend user preferences
For users already familiar with SAP’s ecosystem, tools like SAP Analytics Cloud (SAC), SAP BusinessObjects (BO), and Analysis for Office (AFO) offer an intuitive and deeply integrated experience, allowing them to work seamlessly with SAP data and applications. These tools provide robust analytics and reporting capabilities, tailored specifically to SAP environments, making them ideal for organizations already invested in SAP technologies.
In contrast, hyperscalers typically integrate with non-SAP frontend tools such as Power BI (Azure), QuickSight (AWS), and Looker (Google Cloud), alongside popular third-party tools like Tableau, MicroStrategy, and Qlik. The choice of frontend tools is often driven by industry-leading applications or business user preferences, especially when teams are already skilled in these solutions, enabling flexible analytics across both SAP and non-SAP data environments.
Non-SAP Frontend limitations
When using non-SAP frontends on top of SAP datasets originating from SAP S/4HANA, for example, you may face certain limitations regarding integrations and supported capabilities. Specific SAP functionality may be essential but is not directly supported by non-SAP tools. This may trigger additional development efforts, workarounds, or lack of capabilities. Here are a few examples of such functionality that is natively supported by SAP analytics tools, such as SAC and Analysis for Office:
Master data hierarchies
Time-dependent master data
Query structures used in complex financial reports
Currency/unit conversions
End user language settings
Security & Authorizations
User roles & authorizations
SAP Analytics tools use SAP roles and authorizations, that can be maintained in one environment and then copied/replicated to others, e.g. SAP S/4HANA, SAP BW, or SAC. This can simplify role management for organizations already invested in the SAP ecosystem.
Hyperscalers in combination with the non-SAP frontends provide customizable and granular security features that require separate maintenance, often involving a more complex setup for the hybrid or multi-cloud environments.
5. Cost and Development
Cost efficiency, speed of deployment, and technical skills of your team are other important factors in choosing the right platform.
SAP Business Content
The complexity of SAP ERP data models is effectively addressed through SAP Business Content, a set of pre-configured templates, reports, data models, and best practices designed to accelerate the deployment of SAP solutions. This content is particularly beneficial for systems like SAP BW, SAP S/4HANA, and SAP Analytics Cloud, offering industry-specific tools for streamlined data extraction, process automation, and analytics. By leveraging this content, organizations can significantly reduce development time and adhere to best practices.
In SAP S/4HANA, business content is delivered via CDS views (Core Data Services views), which are virtual data models providing real-time access to complex data representations directly at the database level. These CDS views can be consumed by SAP data warehouses, frontend tools, or even hyperscalers, enabling advanced analytics and reporting across various platforms.
Total Cost of Ownership (TCO) ideas
Hyperscalers operate on a pay-as-you-go model, which can lead to cost savings for organizations with varying workloads. SAP Analytics, on the other hand, might offer a more predictable cost structure for organizations deeply integrated with SAP, avoiding additional expenses related to third-party cloud integration.
Developer profiles: ABAP, HANA vs. SQL, Python
If your development team is skilled in ABAP and HANA, staying within the SAP ecosystem with the SAP Analytics toolset may reduce costs associated with retraining or hiring for new roles. Hyperscalers, with their support for languages like SQL, Python, and modern data science frameworks, are better suited for teams with diverse technical expertise.
Conclusion
The decision between SAP Analytics and hyperscalers depends largely on your organization’s data sources, reporting needs, scalability requirements, frontend preferences, and cost considerations. SAP Analytics tools such as SAP S/4HANA embedded analytics or SAP Analytics Cloud are best suited for organizations heavily invested in SAP systems with a need for real-time, operational reporting. Hyperscalers provide greater scalability, flexibility, and advanced capabilities for organizations that handle diverse data sources and need to scale dynamically.
Often the most effective solution is a hybrid approach, utilizing SAP Analytics tools for core ERP functionalities, operational reporting, business planning, financial consolidation, and hyperscalers for large-scale data processing, integration with cloud and semi-structured data, analytics on streaming sources, APIs, AI services, and advanced analytics.
Comments