AI for SAP Customers: Where to Start?
- Sergei Peleshuk
- Mar 30
- 5 min read
Contents
Artificial Intelligence (AI) is transforming how businesses operate, and SAP customers have a wide range of AI capabilities at their disposal. From automating financial processes to optimizing supply chains and enhancing customer interactions, AI-driven solutions can unlock significant business value. However, selecting the right AI use case and platform can be challenging, requiring a clear understanding of business needs, data availability, and SAP’s AI ecosystem.
This guide provides a structured approach to getting started with AI in SAP, covering industry-specific applications, finance use cases, conversational AI, and a high-level decision tree to help choose the most suitable AI platform.
1. Defining AI Use Cases
SAP customers should begin their AI journey by identifying relevant use cases that align with business objectives, data readiness, and available AI capabilities within their SAP landscape. The first step is to determine whether prebuilt AI solutions in SAP S/4HANA, SAP Analytics Cloud (SAC), SAP Concur, SAP SuccessFactors or other applications meet their needs, or if they require custom AI models. Validate if SAP AI capabilities on SAP BTP can be sufficient for implementing identified use cases.
For more advanced AI scenarios, such as large-scale machine learning, NLP-powered chatbots, and deep learning applications, hyperscalers (AWS, Azure, GCP) provide additional computational power, real-time analytics, and scalability beyond SAP’s native AI offerings.
Measuring AI project's success involves defining clear KPIs, running pilot projects, and scaling AI solutions effectively to drive business value.
Example AI Use Case
Business Challenge | Inaccurate cash flow forecasting affects liquidity planning |
Available Data | Historical transactions, receivables, payables, economic indicators |
AI Capability | Predictive analytics using SAP AI Core & SAP Analytics Cloud |
Success Metrics | 90% forecast accuracy, 15% reduction in working capital requirements |
Pilot Approach | AI model tested on past 12 months of financial data |
Scaling Strategy | Rollout across global finance teams after successful PoC |
2. AI Applications Across Industries
AI is transforming industries by improving efficiency, enhancing decision-making, and elevating customer experience through technologies like machine learning and predictive analytics. Each sector leverages AI differently, from automating processes to optimizing resources and mitigating risks. Below are key AI use cases demonstrating its impact across various industries.
Retail & E-commerce: AI-Driven Customer Experience and Demand Forecasting
Personalized Recommendations
Demand Forecasting
Automated Inventory Management
Fraud Detection in E-commerce
Telecommunications: AI for Network Optimization and Customer Experience
AI-Driven Network Traffic Management
Predictive Maintenance for Telecom Infrastructure
AI-Powered Chatbots and Virtual Assistants
Fraud Detection and Revenue Assurance
Manufacturing: AI-Powered Predictive Maintenance and Quality Control
Predictive Maintenance
Quality Control with Computer Vision
Supply Chain Optimization
Finance & Banking: AI-Enhanced Fraud Detection and Risk Management
Fraud Detection and Prevention
Risk Assessment and Credit Scoring
Automated Regulatory Compliance
Healthcare & Life Sciences: AI for Patient Analytics and Drug Discovery
AI-Powered Patient Analytics
Predictive Disease Outbreak Monitoring
Drug Discovery and Clinical Trials Optimization
Energy & Utilities: AI for Smart Grids and Energy Efficiency
Smart Grid Optimization
Predictive Maintenance for Energy Infrastructure
Renewable Energy Forecasting
3. Use Cases in Corporate Finance
AI is transforming corporate finance by automating routine tasks, improving accuracy, and providing predictive insights that enhance decision-making. From financial forecasting to fraud detection, AI-driven solutions help businesses optimize cash flow, streamline accounting processes, and ensure compliance with regulatory requirements. The following use cases highlight how AI and machine learning are driving efficiency and business value across key finance functions.
1. AI-Powered Financial Forecasting & Planning
Use Case: Predict future revenue, expenses, and cash flow based on historical data and market trends.
2. Automated Invoice Processing & Accounts Payable
Use Case: Reduce manual work in invoice matching, approval workflows, and fraud detection.
3. AI-Driven Cash Flow & Liquidity Management
Use Case: Predict cash inflows and outflows to optimize liquidity.
4. Fraud Detection & Risk Management
Use Case: Identify fraudulent transactions and mitigate financial risks.
5. Intelligent Expense Management
Use Case: Automate expense approvals and detect policy violations.
4. Conversational AI and Chatbots
AI-powered ERP chatbots enable organizations to improve sales, enhance operational efficiency, elevate customer satisfaction, and support real-time decision-making. When integrated with SAP S/4HANA Finance and SAP BTP, these intelligent chatbots automate financial queries, streamline workflows, and optimize user experience. Key finance use cases include:
1. Real-Time Financial Reporting & Insights
Use Case: Executives and finance teams can ask the chatbot for real-time financial statements, balance sheets, or profit & loss summaries.
2. Automated Invoice & Payment Status Tracking
Use Case: Vendors and finance teams can check invoice approvals, due payments, or payment statuses.
3. Intelligent Expense Management & Policy Compliance
Use Case: Employees can submit and track expense claims via chatbot.
The chatbot architecture below enables real-time access to transactional finance or other ERP data, while adhering to predefined chat rules and leveraging a static knowledge base for accurate responses.
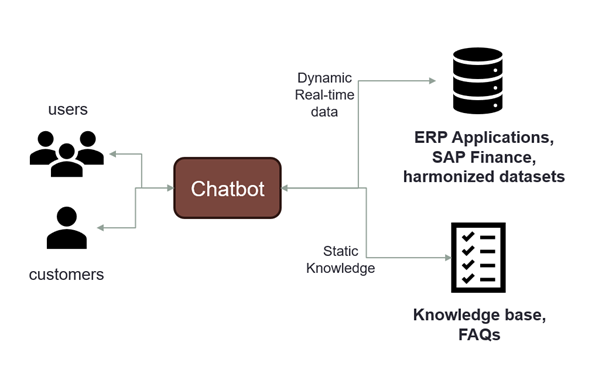
5. Decision Tree for Selecting AI Platform
SAP customers must carefully evaluate their AI needs based on the complexity of their use cases, data processing requirements, and scalability expectations.
While SAP S/4HANA Embedded AI and SAP Analytics Cloud (SAC) offer built-in AI for predictive accounting, forecasting, and anomaly detection (varies for S/4 cloud vs. on-premise), more advanced scenarios require SAP AI Core, AI Foundation (BTP), and SAP Business Data Cloud (BDC) for custom AI models.
For large-scale AI workloads such as NLP, image recognition, and deep learning, hyperscalers (AWS, Azure, GCP) provide the computational power and flexibility needed to extend AI capabilities beyond SAP's native solutions.
Use Case | AI Requirement | Best AI Platform |
• Predictive Accounting • Cash Flow Forecasting • Automated Invoice Processing • Smart GR/IR Reconciliation | Standard AI in SAP business processes | SAP S/4HANA Embedded AI |
• Sales & Demand Forecasting • Anomaly Detection • Predictive Maintenance • Financial Planning & Analysis | Predictive analytics & forecasting | SAP Analytics Cloud (SAC) AI |
• AI-Powered Document Processing • Intelligent Chatbots • ML-Based Risk Scoring, classification • AI for Manufacturing Quality Control • AI-Based IoT Anomaly Detection • AI for Regulatory Compliance • AI-Driven HR Analytics | Custom AI models for SAP workflows | SAP AI Core & AI Foundation (BTP), SAP Business Data Cloud (BDC), Hyperscalers |
• AI-Based Image & Video Recognition • Natural Language Processing (NLP) • Large-Scale Fraud Detection • AI-Powered Market & Sentiment Analysis | Large-scale AI & ML on cloud | Hyperscalers (AWS, Azure, GCP) |
Choosing the right AI platform depends on the specific business use case, AI complexity, harmonization of data sources and integration requirements within the SAP ecosystem. This decision tree helps SAP customers identify the most suitable AI solution, from embedded AI in SAP S/4HANA for standard automation to hyperscalers for large-scale AI and machine learning applications.
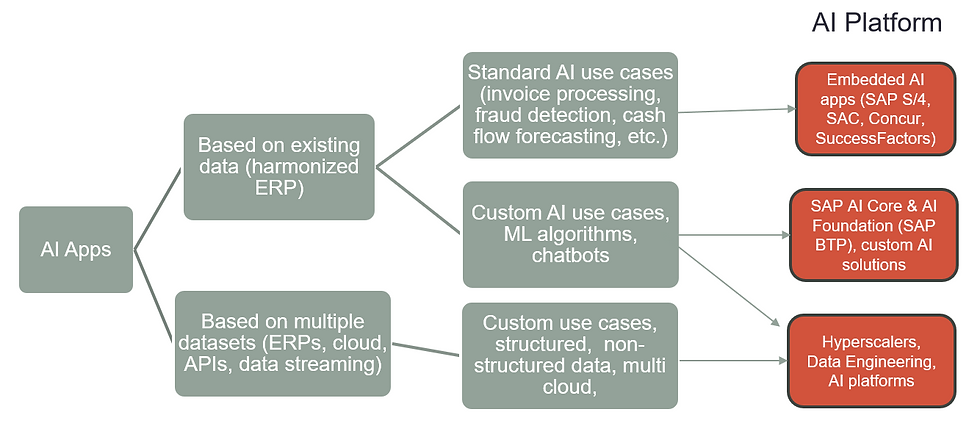
AI offers SAP customers powerful capabilities to automate processes, enhance decision-making, and drive business innovation across industries. Choosing the right AI solution depends on business needs, data readiness, availability of relevant skills and appropriate SAP or hyperscaler platforms.
By following a structured approach: defining AI use cases, exploring industry applications, leveraging conversational AI, and using the decision tree for platform selection businesses can successfully integrate AI into their SAP landscape and maximize its value.
Comments